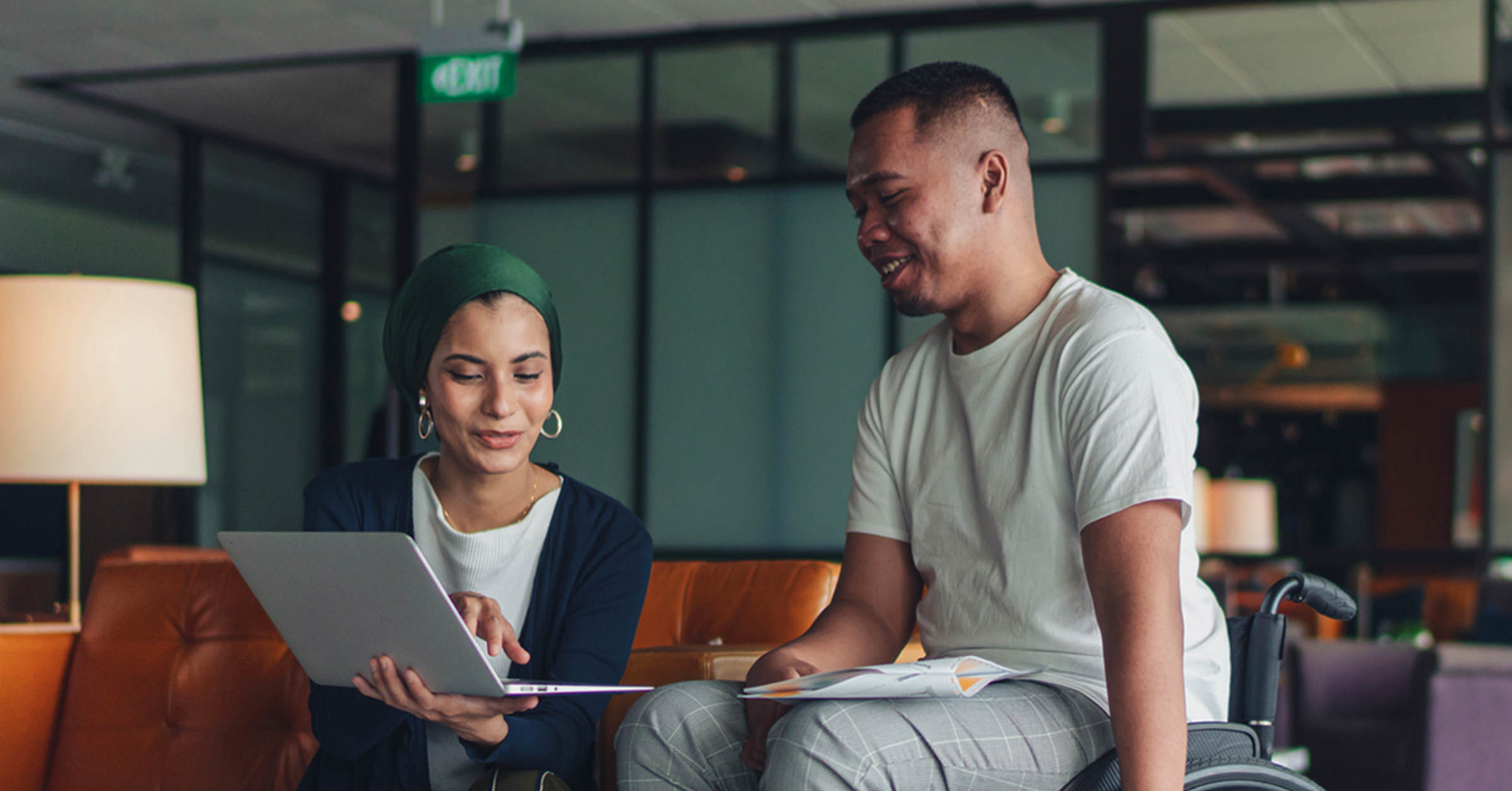
A Learner-Centric Approach for Scalable Success
People are continuously questioning the purpose of obtaining a college degree, as well as its benefits. This recurring problem is not about anyone opposing student success; it is about perspective. We must all share the same definition of success, in all of its varied manifestations.
For example, a student who enrolls in a university to study engineering discovers that they dislike the subject and transfers to another school. Is this outcome a success even though it has a negative impact on the original school's retention rate?
From an institution-centric perspective, it appears that the institution failed that student. Schools are held accountable based upon aggregated rates of student success, such as their retention and job placement rates. For individual students, though, these are all or nothing measures. They either graduate or not, get a job or not. A 97% job placement rate is very impressive for a school, but what about a student who comprises part of the remaining 3%? Students may feel like "just a number" if educators only concentrate on the strategic, aggregate definitions, without providing them with individual attention or the chance to personalize their learning.
A learner-centric approach can help serve both interests. It can fulfill the altruistic expression of institutional mission statements and drive relational experiences with students while also meeting the aggregate demands of accrediting bodies and government agencies.
Retention rates are changed one student at a time. Every time our efforts make a difference for one student, it positively impacts the broader retention demands. Big data and analytics can be used to ensure that students aren't treated as numbers. We can scale personalization and learner-centricity by democratizing data through early alert systems, non-cognitive data, and labor market data.
Democratizing Data
Democratized data empowers individual users with access to the same data as central authorities. There needs to be a healthy balance between certain safeguards and ensuring all members of your institution have access to appropriate data at the right time so they can make the best decisions for their students.
The chief difference between the student success data a VP of enrollment would consider and the data an advisor would use is in the level of aggregation. The VP level will likely leverage these data in aggregate to make the broadest strategic impact. The advisor can take more tactical action, prioritizing outreach and intervention. Even learners themselves can leverage analytics to make data-informed decisions that reflect their autonomy and self-actualization while also strengthening the unique bond between them and the institution.
Non-Cognitive Data
Non-cognitive data has long been advocated for in student success and retention literature. These data, whether on student motivation, self-efficacy, resilience, or dozens of other constructs, assist institutions in serving the whole studenti. Senior leaders can use aggregate data from these sources to develop appropriate curricula, such as first-year experiencesii. Tactically, advisors can use this information to reach out to and assist those who are most in need.
With appropriate guidance, learners can harness these data to inform future decisions. Consider strengths-based inventories, where students develop a fundamental understanding of themselves and the unique value they bring to any group setting. This fosters a sense of identity and self-efficacy which subsequently emboldens retention and graduation efforts while also appealing to employers.
Early Alert Systems
Early warning systems are another emerging area of analytics in the student success space. These are frequently based on a plethora of data sets. Attendance data, for example, can be aggregated and reported to external stakeholders such as Return to Title IV funds (R2T4) to establish clear strategic guidelines for engagement policies. Advisors can use this information to prioritize outreach and intervention, often detecting student risk before it is obvious.
Sharing with learners themselves provides a new level of agency and control, as “simply making students aware that they are at risk…motivates them to seek help and change their academic behaviour”iii. Once again, a simple set of data, shared across myriad stakeholders whom all embrace learner-centricity, amplifies the efforts of each stakeholder.
Labor Market Data
Each degree bears a multitude of learning outcomes, but students often miss the ability to articulate those outcomes into tangible skills. Certain degrees are more straightforward than others. A student who wants to be a nurse will almost certainly pursue a nursing degree. But what about students who do not achieve upper-division status, do not meet practicum requirements, or choose to change careers? It is difficult to position those specific skills in the context of a broader labor market.
As the labor market changes, career services teams can use these labor market data to assist their learners in creating dynamic and effective resumes. Career services teams can assist their learners in creating sophisticated and compelling resumes that are responsive to an ever-changing labor market.
Furthermore, having these data in the hands of a learner empowers action. Students can select a course that is not only interesting but also fills a skill gap. Showcasing and articulating these developed skills epitomizes a learner-centric approach that is fueled by data.
Conclusion
To ensure that our students achieve self-actualization through meaningful learning experiences, we must develop a shared understanding of what success looks like for them. We then must share these crucial data insights with learners so they can make wise decisions and champion their successiv.
Higher education leaders can be proactive in this pursuit by promoting innovation through a learner-centric, data-informed strategy. Doing so will demonstrate the superior value of a postsecondary education and will prepare your students for success now and in the future.
References
Credé, M., Roch, S. G., & Kieszczynka, U. M. (2010). Class attendance in college: A meta-analytic review of the relationship of class attendance with grades and student characteristics. Review of Educational Research, 80(2), 272-295.
Lane, F. C., & Miller, A. P. (2019). First-Year Seminars: A Comparison of Course Characteristics and High Impact Practices at Two-Year Colleges.
Jayaprakash, S. M., Moody, E. W., Lauría, E. J., Regan, J. R., & Baron, J. D. (2014). Early alert of academically at-risk students: An open source analytics initiative. Journal of Learning Analytics, 1(1), 6-47.
Almond-Dannenbring, T., Easter, M., Feng, L., Guarcello, M., Ham, M., Machajewski, S., Maness, H., Miller, A.P., Mooney, S., Moore, A., Kendall, E. (2022). A Framework for Student Success Analytics. EDUCAUSE. Retrieved from: https://library.educause.edu/resources/2022/5/a-framework-for-student-success-analytics