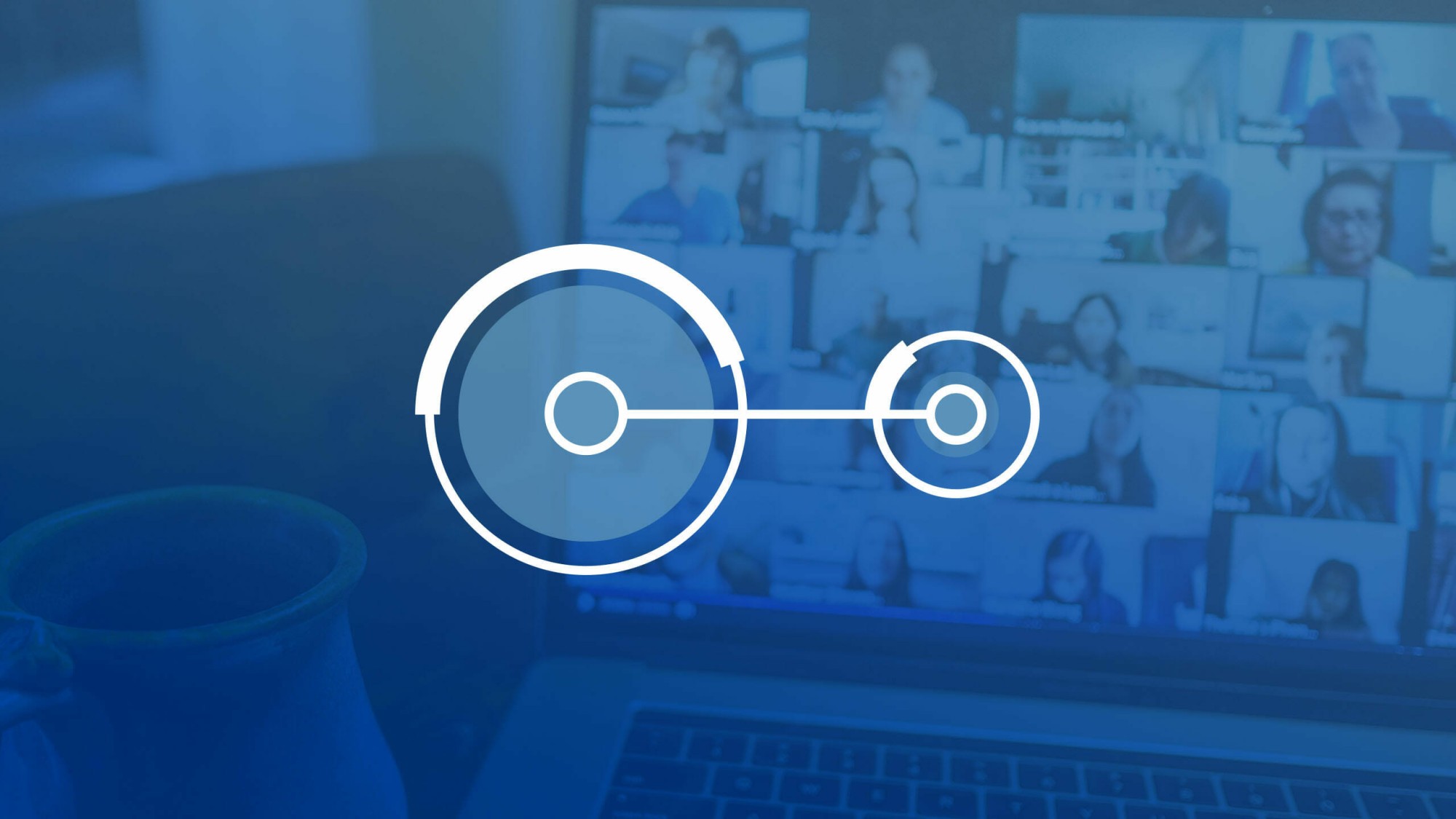
Comparing Course Evaluation Qualitative Data During COVID-19
This content was previously published by Campus Labs, now part of Anthology. Product and/or solution names may have changed.
In a recent blog post, Comparing Course Evaluation Quantitative Data During COVID-19, quantitative data collected from course evaluations conducted in Spring 2020 were compared with those in Spring 2019. We found no meaningful differences in student ratings of teaching, the course, and self-reported progress on course-relevant learning objectives. For this blog post, qualitative data from student written comments were compared across the two-year period. Similar to the quantitative results, we found no meaningful differences in the average word count, proportions of negative and positive words, content of word clouds, sentiments, linguistic complexity, and readability.
We first split students’ written comments into tokens, which are meaningful units of text, such as single words, that served as a unit of analysis. As the following table shows, despite the challenges instructors and students faced this past spring, summary statistics were not meaningfully different from 2019. The average word counts, which provide a proxy for how much students cared, were very similar. Moreover, the proportions of positive and negative words, which reveal students’ general feelings, were identical.
We then did a count of the most frequent positive and negative words. The bar charts below show that the most frequent positive and negative words were basically the same across the two years.
To get a bigger picture of the range of words used, we then created word clouds of the top 100 positive and negative words. Each of the word clouds below position negative words at the top and positive words at the bottom. They reveal remarkable similarities between 2019 and 2020.
However, text polarity (the degree of negativity and positivity) is just one dimension of text analysis. To go deeper, we conducted sentiment analysis at the sentence level, which goes beyond detecting positive, negative or neutral opinions. We specifically employed sentimentr (Rinker, 2017), which attempts to control for valence shifters, which are words or phrases that affect (e.g., negate, intensify, diminish) polarity. Examples include negators, which flip the sign of a polarized word (e.g., “I do not like it.”); amplifiers, which increase the impact of a word (e.g., “I really like it.”); de-amplifiers, which reduce the impact of a word (e.g., “I hardly like it.”); and adversative conjunctions, which overrule a previous clause containing a polarized word (e.g., “I like it but it’s not worth it.”). Valence shifters matter because they can reverse or overrule the sentiment of an entire clause. So, if they occur fairly frequently, a simple dictionary search of positive and negative words may not reveal a student’s intended sentiments.
In looking at the table below, mean values for sentiment were nearly identical across the two years, which indicates that students’ intended feelings about teaching and the course were slightly positive in both 2019 and 2020. In the same way, mean scores on diversity—a measure of linguistic skill or the complexity of ideas--were very similar. Finally, readability was at about the 7th grade level in both years.
So, as did the quantitative analyses reported previously, the current results reveal notable similarities between 2019 and 2020 course evaluations, even though many instructors had never taught online and many students had never taken an online course. That we found no meaningful differences in the polarity and sentiments expressed in student course evaluations is perhaps unexpected. Nonetheless, it speaks to the gallant efforts administrators, faculty, and students put forth in the face of a very challenging semester.
Source:
Rinker, T. W. (2019). sentimentr: Calculate Text Polarity Sentiment
version 2.7.1. http://github.com/trinker/sentimentr
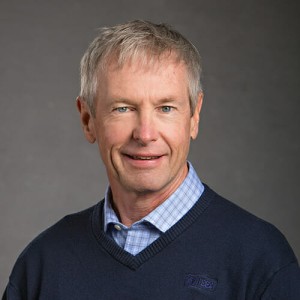
Steve Benton, Ph.D.
Steve Benton, Ph.D., is a data scientist in the Campus Labs data science team. Previously, he was Senior Research Officer at The IDEA Center where, from 2008 to 2019, he led a research team that designed and conducted reliability and validity studies for IDEA products. He is also Emeritus Professor and Chair of Special Education, Counseling, and Student Affairs at Kansas State University where he served from 1983 to 2008. His areas of expertise include student ratings of instruction, teaching and learning, and faculty development and evaluation. Steve received his Ph.D. in Psychological and Cultural Studies from the University of Nebraska-Lincoln, from whom he received the Alumni Award of Excellence in 1997. He is a Fellow in the American Psychological Association and the American Educational Research Association.